TBA
November, 25th, 2020
TITLE
Donnez plus de détails sur vos services. Utilisez cette mise en page répétitive pour afficher votre contenu. C'est le moyen idéal pour tenir vos clients informés. Vous souhaitez personnaliser ce texte à votre image ? Glissez-déposez simplement vos éléments comme des images, des liens ou du texte ou connectez les données de votre collection. Donnez plus de détails sur vos services. Utilisez cette mise en page répétitive pour afficher votre
TBA
November, 25th, 2020
TITLE
Donnez plus de détails sur vos services. Utilisez cette mise en page répétitive pour afficher votre contenu. C'est le moyen idéal pour tenir vos clients informés. Vous souhaitez personnaliser ce texte à votre image ? Glissez-déposez simplement vos éléments comme des images, des liens ou du texte ou connectez les données de votre collection. Donnez plus de détails sur vos services. Utilisez cette mise en page répétitive pour afficher votre
TBA
November, 18, 2020
TITLE
Donnez plus de détails sur vos services. Utilisez cette mise en page répétitive pour afficher votre contenu. C'est le moyen idéal pour tenir vos clients informés. Vous souhaitez personnaliser ce texte à votre image ? Glissez-déposez simplement vos éléments comme des images, des liens ou du texte ou connectez les données de votre collection. Donnez plus de détails sur vos services. Utilisez cette mise en page répétitive pour afficher votre
TBA
November, 25th, 2020
TITLE
Donnez plus de détails sur vos services. Utilisez cette mise en page répétitive pour afficher votre contenu. C'est le moyen idéal pour tenir vos clients informés. Vous souhaitez personnaliser ce texte à votre image ? Glissez-déposez simplement vos éléments comme des images, des liens ou du texte ou connectez les données de votre collection. Donnez plus de détails sur vos services. Utilisez cette mise en page répétitive pour afficher votre
TBA
November, 25th, 2020
TITLE
Donnez plus de détails sur vos services. Utilisez cette mise en page répétitive pour afficher votre contenu. C'est le moyen idéal pour tenir vos clients informés. Vous souhaitez personnaliser ce texte à votre image ? Glissez-déposez simplement vos éléments comme des images, des liens ou du texte ou connectez les données de votre collection. Donnez plus de détails sur vos services. Utilisez cette mise en page répétitive pour afficher votre
TBA
November, 18, 2020
TITLE
Donnez plus de détails sur vos services. Utilisez cette mise en page répétitive pour afficher votre contenu. C'est le moyen idéal pour tenir vos clients informés. Vous souhaitez personnaliser ce texte à votre image ? Glissez-déposez simplement vos éléments comme des images, des liens ou du texte ou connectez les données de votre collection. Donnez plus de détails sur vos services. Utilisez cette mise en page répétitive pour afficher votre
TBA
November, 18, 2020
TITLE
Donnez plus de détails sur vos services. Utilisez cette mise en page répétitive pour afficher votre contenu. C'est le moyen idéal pour tenir vos clients informés. Vous souhaitez personnaliser ce texte à votre image ? Glissez-déposez simplement vos éléments comme des images, des liens ou du texte ou connectez les données de votre collection. Donnez plus de détails sur vos services. Utilisez cette mise en page répétitive pour afficher votre
Karel Svoboda
Allen Institute
October 30, 2024

VVTNS Fifth Season Opening Lecture​
​
Illuminating synaptic learning​​​
How do synapses in the middle of the brain know how to adjust their weight to advance a behavioral goal (i.e. learning)? This is referred to as the synaptic ‘credit assignment problem’. A large variety of synaptic learning rules have been proposed, mainly in the context of artificial neural networks. The most powerful learning rules (e.g. back-propagation of error) are thought to be biologically implausible, whereas the widely studied biological learning rules (Hebbian) are insufficient for goal-directed learning. I will describe ongoing work focused on understanding synaptic learning rules in the cortex in a brain-computer interface task.
Hannah Choi
Georgia Tech
November 6, 2024
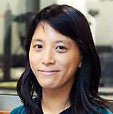
Unraveling information processing through functional networks
While anatomical connectivity changes slowly through synaptic learning, the functional connectivity of neurons changes rapidly with ongoing activity of neurons and their functional interactions. Functional networks of neurons and neural populations reflect how their interactions change with behaviors, stimulus types, and internal states. Therefore, the information propagation across a network can be analyzed through the varying topological properties of the functional networks. Our study investigates the functional networks of the visual cortex at both the single-cell and population levels. Our analyses of functional connectivity of single neurons, constructed from spiking activity in neural populations of the visual cortex, reveal local and global network structures shaped by stimulus complexity. In addition, we propose a new method for inferring functional interactions between neural populations that preserves biologically constrained anatomical connectivity and signs. Applying our method to 2-photon data from the mouse visual cortex, we uncover functional interactions between cell types and cortical layers, suggesting distinct pathways for processing expected and unexpected visual information.
Friedemann Zenke
University of Basel
November 13, 2024
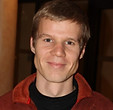
Learning invariant representations through prediction
Discriminating distinct objects and concepts from sensory stimuli is essential for survival. Our brains perform this processing in deep sensory networks shaped through plasticity. However, our understanding of the underlying plasticity mechanisms remains rudimentary. I will introduce Latent Predictive Learning (LPL), a plasticity model prescribing a local learning rule that combines Hebbian elements with predictive plasticity. I will show that deep neural networks equipped with LPL develop disentangled object representations without supervision. The same rule accurately captures neuronal selectivity changes observed in the primate inferotemporal cortex in response to altered visual experience. Finally, our model generalizes to spiking neural networks and naturally accounts for several experimentally observed properties of synaptic plasticity, including metaplasticity and spike-timing-dependent plasticity (STDP). LPL thus constitutes a plausible normative theory of representation learning in the brain while making concrete testable predictions.

The algebra of cognition
Chris Eliasmith
University of Waterloo November 20, 2024
In recent years, my lab and others have demonstrated the value of vector symbolic algebras (VSAs) for capturing a wide variety of neural and behavioural results. In this talk I discuss the surprising and compelling variety of tasks and styles of reasoning that are well-suited to descriptions using a specific VSA. These tasks include path integration, navigation, Bayesian reasoning, sampling, memorization, and logical inference. The resulting spiking neural network models capture various hippocampal cell types (grid, place, border, etc.), behavioural errors, and a variety of observed neural dynamics.

Memming Park
Champalimaud Foundation
November 27, 2024
Back to the Continuous Attractor
Continuous attractors offer a unique class of solutions for storing continuous-valued variables in recurrent system states for indefinitely long time intervals. Unfortunately, continuous attractors suffer from severe structural instability in general---they are destroyed by most infinitesimal changes of the dynamical law that defines them. This fragility limits their utility especially in biological systems as their recurrent dynamics are subject to constant perturbations. We observe that the bifurcations from continuous attractors in theoretical neuroscience models display various structurally stable forms. Although their asymptotic behaviors to maintain memory are categorically distinct, their finite-time behaviors are similar. We build on the persistent manifold theory to explain the commonalities between bifurcations from and approximations of continuous attractors. Fast-slow decomposition analysis uncovers the existence of a persistent slow manifold that survives the seemingly destructive bifurcation, relating the flow within the manifold to the size of the perturbation. Moreover, this allows the bounding of the memory error of these approximations of continuous attractors. Finally, we train recurrent neural networks on analog memory tasks to support the appearance of these systems as solutions and their generalization capabilities. Therefore, we conclude that continuous attractors are functionally robust and remain useful as a universal analogy for understanding analog memory.
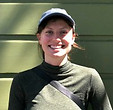
Task structure shapes underlying dynamical systems
that implement computation
The talk will be in two parts. 1) First, I will present published work on multitasking artificial recurrent neural networks that revealed "dynamical motifs". Dynamical motifs are recurring patterns of network activity that implement specific computations through dynamics, such as attractors, decision boundaries and rotations. Motifs are reused across tasks and reflect the modular subtask structure of commonly studied cognitive tasks. We believe this compositional structure to be a feature of most complex cognitive tasks. This work establishes dynamical motifs as a fundamental unit of compositional computation, intermediate between neuron and network. 2) Building on these insights, we are now investigating whether shared dynamical motifs can explain the effectiveness of curriculum learning in animal behavior. Also known as animal shaping, curriculum learning is a training approach where complex tasks are learned in stages through a series of subtasks. Our work provides a novel framework for analysis of neural and behavioral data and has the potential to guide the design of optimal training protocols for both artificial systems and for animals.
Laura Driscoll
Allen Institute
December 4, 2024
Noam Sadon-Grosman
ELSC, The Hebrew University of Jerusalem​
December 11, 2024
Somatomotor to Higher Order Cognition -
The Detailed Organization of the Human Cerebellum
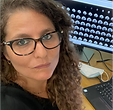
The cerebellum is long known for its somatomotor functions. Recent evidence has converged to suggest that major portions of the human cerebellum are linked to cognitive and affective functions. In this talk, I will present new insights into the functional organization of the human cerebellum. Our findings reveal three distinct somatomotor representations, including a newly identified third map that is spatially dissociated from the two well-established body representations. Between these body representations, a large megacluster extending across Crus I/II was consistently found with subregions linked to higher-order cerebral association networks. Within this megacluster, specific regions responded to domain-flexible cognitive control, while juxtaposed regions differentially responded to language, social, and spatial/episodic task demands. Similarly organized clusters also exist in the caudate consistent with the presence of multiple basal ganglia–cerebellar–cerebral cortical circuits that maintain functional specialization across their entire distributed extents.
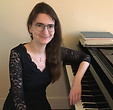
Claire Meissner-Bernard
Friedrich Miescher Institute
for biomedical research
Basel
December 18, 2024
Properties of memory networks with excitatory-inhibitory assemblies​​
Classical views suggest that memories are stored in assemblies of excitatory neurons that become strongly interconnected during learning. However, recent experimental and theoretical results have challenged this view, leading to the hypothesis that memories are encoded in assemblies containing both excitatory (E) and inhibitory (I) neurons. Understanding the effects of these E-I assemblies on memory function is therefore essential. Using a biologically constrained model of an olfactory memory network, I will first describe how introducing E-I assemblies reorganizes odor-evoked activity patterns in neural state space. Indeed, the “geometry” of neural activity provides valuable insights about the computational properties of neural networks. I will then describe the behavior of networks with E-I assemblies upon partial manipulation of inhibitory neurons. Finally, I will discuss recent experimental data supporting predictions of the model.
​
December 25, 2024
Merry Christmas
​
January 1, 2025
Happy New Year
Dmitry Krotov
IBM Research, Cambridge
USA
January 8, 2025
VVTNS New Year Opening Lecture
​
Dense Associative Memory and its potential role in brain computation
​​​
.jpeg)
Dense Associative Memories (Dense AMs) are energy-based neural networks that share many desirable features of celebrated Hopfield Networks but have superior information storage capabilities. In contrast to conventional Hopfield Networks, which were popular in the 1980s, DenseAMs have a very large memory storage capacity - possibly exponential in the size of the network. This aspect makes them appealing tools for many problems in AI and neurobiology. In this talk I will describe two theories of how DenseAMs might be built in biological “hardware”. According to the first theory, DenseAMs arise as effective theories after integrating out a large number of neuronal degrees of freedom. According to the second theory, astrocytes, a particular type of glia cells, serve as core computational units enabling large memory storage capabilities. This second theory challenges a common point of view in the neuroscience community that astrocytes play the role of only passive house-keeping support structures in the brain. In contrast, it suggests that astrocytes might be actively involved in brain computation and memory storage and retrieval. This story is an illustration of how computational principles originating in physics may provide insights into novel AI architectures and brain computation.
Jonathan Pillow
Princeton University
January 15, 2025
New methods for tracking and control of dynamic animal behavior during learning

The dynamics of learning in natural and artificial environments is a problem of great interest to both neuroscientists and artificial intelligence experts. However, standard analyses of animal training data either treat behavior as fixed, or track only coarse performance statistics (e.g., accuracy and bias), providing limited insight into the dynamic evolution of behavioral strategies over the course of learning. To overcome these limitations, we propose a dynamic psychophysical model that efficiently tracks trial-to-trial changes in behavior over the course of training. In this talk, I will describe recent work based on a dynamic logistic regression model that captures the time-varying dependencies of behavior on stimuli and other task covariates, which we applied to mouse training data from the International Brain Lab (IBL). Secondly, I will discuss efforts to infer animal learning rules from time-varying behavior in order to characterize how they adjust their policy in response to reward. Finally, I will describe recent work on adaptive optimal training, which combines ideas from reinforcement learning and adaptive experimental design to formulate methods for inferring animal learning rules from behavior, and using these rules to speed up animal training.
Srdjan Ostojic
ENS, Paris
January 22, 2025
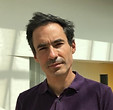
Structured Excitatory-Inhibitory Networks: a low-rank approach
Networks of excitatory and inhibitory (EI) neurons form a canonical circuit in the brain. Classical theoretical analyses of dynamics in EI networks have revealed key principles such as EI balance or paradoxical responses to external inputs. These seminal results assume that synaptic strengths depend on the type of neurons they connect but are otherwise statistically independent. However, recent synaptic physiology datasets have uncovered connectivity patterns that deviate significantly from independent connection models. Simultaneously, studies of task-trained recurrent networks have emphasized the role of connectivity structure in implementing neural computations. Despite these findings, integrating detailed connectivity structures into mean-field theories of EI networks remains a substantial challenge. In this talk, I will outline a theoretical approach to understanding dynamics in structured EI networks by employing a low-rank approximation based on an analytical computation of the dominant eigenvalues of the full connectivity matrix. I will illustrate this approach by investigating the effects of pair-wise connectivity motifs on linear dynamics in EI networks. Specifically, I will present recent results demonstrating that an over-representation of chain motifs induces a strong positive eigenvalue in inhibition-dominated networks, generating a potential instability that challenges classical EI balance criteria. Furthermore, by examining the effects of external input, we found that chain motifs can, on their own, induce paradoxical responses, wherein an increased input to inhibitory neurons leads to a counterintuitive decrease in their activity through recurrent feedback mechanisms. Altogether, our theoretical approach opens new avenues for relating recorded connectivity structures with dynamics and computations in biological networks.
A Geometric Approach for the Study of
Functional Connectivity Dynamics
Hadas Benisty
Technion
January 29, 2025
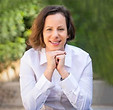
Functional connectivity has been the focus of many research groups aiming to study the interaction between cells and brain regions. A standard method for analyzing connectivity is to statistically compare pairwise interactions between cells or brain regions across behavioral states or conditions. This methodology ignores the intrinsic properties of functional connectivity as a multivariate and dynamic signal, expressing the correlational configuration of the network. In this talk, I will present a geometric approach, combining Graph Theory and Riemannian Geometry to build "a graph of graphs" and extract the latent dynamics of the overall correlational structure. Using this approach, we formulate the statistical relations between network dynamics and spontaneous behavior as a second-order Taylor’s expansion. Our analysis shows that fast fluctuations in functional connectivity of large-scale cortical networks are closely linked to variations in behavioral metrics related to the arousal state. We further expand this methodology to longer time scales to study the effect of dopamine on network dynamics in the primary motor cortex (M1) during learning. We developed a series of analysis methods indicating that as animals learn to perform a motor task, the network of pyramidal neurons in layer 2-3 gradually and monotonically reorganizes toward an "expert" configuration. Our results highlight the critical role of dopamine in driving synaptic plasticity: Blocking dopaminergic neurotransmission locally in M1 prevented motor learning at the behavioral level and concomitantly halted plasticity changes in network activity and in functional connectivity.
Matthew Golub
University of Washington
February 5, 2025
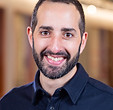
Active learning of neural population dynamics
Recent advances in techniques for monitoring and perturbing neural populations have greatly enhanced our ability to study circuits in the brain. In particular, two-photon holographic optogenetics now enables precise photostimulation of experimenter-specified groups of individual neurons, while simultaneous two-photon calcium imaging enables the measurement of ongoing and induced activity across the neural population. Despite the enormous space of potential photostimulation patterns and the time-consuming nature of photostimulation experiments, very little algorithmic work has been done to determine the most effective photostimulation patterns for identifying the neural population dynamics. Here, I will discuss ongoing development of active learning techniques to efficiently select which neurons to stimulate such that the resulting neural responses will best inform a dynamical model of the neural population activity.
Lea Duncker
Columbia University
February 12, 2025

Evaluating dynamical systems hypotheses for pattern generation
in motor cortex
The rich repertoire of skilled mammalian behavior is the product of neural circuits that generate robust and flexible patterns of activity distributed across populations of neurons. Decades of associative studies have linked many behaviors to specific patterns of population activity, but association alone cannot reveal the dynamical mechanisms that shape those patterns. Are local neural circuits high-dimensional dynamical reservoirs able to generate arbitrary superpositions of patterns with appropriate excitation? Or might circuit dynamics be shaped in response to behavioral context so as to generate only the low-dimensional patterns needed for the task at hand? Here, we address these questions within primate motor cortex by delivering optogenetic and electrical microstimulation perturbations during reaching behavior. We develop a novel analytic approach that relates measured activity to theoretically tractable, dynamical models of excitatory and inhibitory neurons. Our computational modeling framework allows us to quantitatively evaluate different hypotheses about the dynamical mechanisms underlying pattern generation against perturbation responses. Our results demonstrate that motor cortical activity during reaching is shaped by a self-contained, low-dimensional dynamical system. The subspace containing task-relevant dynamics proves to be oriented so as to be robust to strong non-normal amplification within cortical circuits. This task dynamics space exhibits a privileged causal relationship with behavior, in that stimulation in motor cortex perturbs reach kinematics only to the extent that it alters neural states within this subspace. Our results resolve long-standing questions about the dynamical structure of cortical activity associated with movement, and illuminate the dynamical perturbation experiments needed to understand how neural circuits throughout the brain generate complex behavior
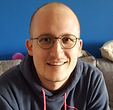
Representational drift reflects ongoing balancing of stochastic changes by Hebbian learning
Even in stable environments, sensory responses undergo continuous reformatting, a phenomenon known as representational drift. Using chronic calcium imaging in mouse auditory cortex, we show that during this representational drift signal correlations predict future noise correlations, suggesting that stimulus-driven co-activation strengthens effective connectivity via Hebbian-like plasticity. Linear network models reveal that these temporal dependencies between signal and noise correlations emerge only when Hebbian learning balances stochastic synaptic changes, preventing functional degradation. Our findings highlight how ongoing input-driven plasticity stabilizes neural representations amidst inherent synaptic variability.
Songting Li
Shanghai Jiao Tong University
February 26, 2025
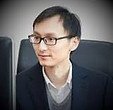
Timescale localization and signal propagation
in the large-scale cortical network
In the brain, while early sensory areas encode and process external inputs rapidly, higher-association areas are endowed with slow dynamics to benefit information accumulation over time. This property raises the question of why diverse timescales are well localized rather than being mixed up across the cortex, despite high connection density and an abundance of feedback loops that support reliable signal propagation. In this talk, we will address this question by analyzing a large-scale network model of the primate cortex, and we identify a novel dynamical regime termed "interference-free propagation". In this regime, the mean components of the synaptic currents to each downstream area are imbalanced to ensure signals to propagate reliably, while the temporally fluctuating components of the synaptic inputs governed by upstream areas' timescales are largely canceled out, leading to the localization of its own timescale in each downstream area. Our result provides new insights into the operational regime of the cortex, leading to the coexistence of hierarchical timescale localization and reliable signal propagation.
Yonatan Loewenstein
ELSC, The Hebrew University
March 5, 2025
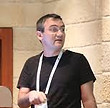
On Idiosyncratic Biases in Decision-Making
Why do individuals, both humans and animals, exhibit personal biases in two-alternative decision-making tasks, even when no clear reason exists to favor one alternative over another? In this talk, I will explore two competing hypotheses to explain these idiosyncratic biases. The first suggests that such tendencies arise from unique personal experiences, where past associations between actions and feedback influence future choices. The second hypothesis proposes that the bias reflects irreducible microscopic heterogeneities in the dynamics of decision-making networks. I will present experimental data and theoretical findings that support the latter hypothesis, shedding new light on the neural mechanisms behind seemingly irrational preferences.
Olivier Marre
Institut de la Vision, Paris
March 12, 2025
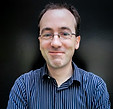
A perturbative approach to understand retinal computations
A major challenge in sensory systems is to understand how neurons extract information from the natural environment. Models derived from their responses to artificial stimuli often have a hard time to generalize and predict responses to natural scenes. However, models directly learned on the responses to natural scenes can be hard to interpret. To address this issue, we have recently developed an approach where we add small perturbations to natural scenes and measure how these perturbations change neuronal responses, to better understand the features extracted by sensory neurons. I will show several applications of this approach in the retina, and how it allowed us to uncover non-linear computations performed by ganglion cells, the retinal output.
Marcelo Rozenberg
CNRS, Paris
March 19, 2025
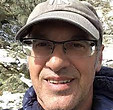
Dynamics of neural motifs realized with a minimal memristive neuro-synaptic unit
The use of electronic circuits to model neural systems goes back to C. Mead and is present in models, from leaky-integrate-and-fire to Hodking-Huxley. Simulating neural network with analog hardware is attractive: it allows to implement neurocomputations in real time without discretization approximations, it has perfect simulation-time scaling with system size, and it provides ready-to-deploy neuromorphic circuit for applications. There are implementations in CMOS technology, however, they are complex, require sophisticated fabrication facilities and, most important, suffer from significant device mismatch. In a radically different approach, based on the concept of memristors, we introduce a neuro-synaptic circuit of unprecedented simplicity, with readily available cheap off-the-shelf electronic components, that can quantitatively reproduce textbook theoretical neuron and synaptic current models. Our neuron circuits can avoid the mismatch problem and are easily tuneable at bio-compatible time-scales. We first introduce a voltage-gated conductance bursting neuron model that produces spike traces that bare striking similarity to experimental recordings. We then introduce synaptic current circuits and show the modularity of our method implementing neurocomputing primitives of basic network motifs, including CPGs. With this "theoretical hardware" approach we show: (i) that neuron adaptation and self-excitation can be viewed as a self-consistent dynamical problem; (ii) that a dynamical memory can be minimally implemented with a single recursive spiking neuron; (iii) that an adaptive membrane current reveals a connection between bursting and the driven harmonic oscillator, perhaps pointing to a neural correlate of the pendular limb motion. Finally we discuss the limitation of the approach to networks of mid-size and its potential application for brain-machine-interfaces, robotics and AI.
Eve of Cosyne
No Seminar
March 26, 2025
The following day of Cosyne​
No Seminar
April 2, 2025
James DiCarlo
MIT
April 9, 2025
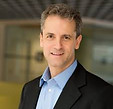
CARL VAN VREESWIJK MEMORIAL LECTURE 2025
​
Do contemporary, machine-executable models of primate sensory systems unlock the ability to non-invasively, beneficially modulate high level brain states?
​
Over the past decade, neuroscience, cognitive science and computer science (“AI”) converged to create specific, image-computable, deep neural network models intended to appropriately abstract, emulate and explain the mechanisms of primate ventral visual processing, up to its deepest neural level, the inferior temporal cortex (IT). Because these leading neuroscientific emulation models — aka “digital twins” — are fully observable and machine-executable, they offer predictive and potential application power that our field’s prior conceptual models did not. Our team’s ongoing work is aimed at asking if current digital twin models might support non-invasive, beneficial brain modulation. In this talk, I will describe a key result: we demonstrate that we can use a digital twin to design spatial patterns of light energy that, when “added” to the organism’s retinal input in the context of ongoing natural visual processing, results in precise modulation (i.e. rate bias) of the pattern of a population of IT neurons (where any intended modulation pattern is chosen ahead of time by the scientist). Because the IT visual neural populations are known to directly connect to and modulate downstream neural circuits (e.g. amygdala) that may underlie psychological affective states (e.g. mood and anxiety), this novel basic science may unlock a new, non-invasive application avenue of potential future human clinical benefit. This progress and new impact possibilities resulted from convergent brain science and AI engineering efforts in the domain of visual object intelligence. I will motivate this as just one example of what I believe will unlock in other domains of human intelligence as brain scientists and AI engineers collaborate to develop machine-executable models of the underlying mechanisms of those still-mysterious domains.
​
April 16, 2025
No Seminar
​
Easter/Passover vacation